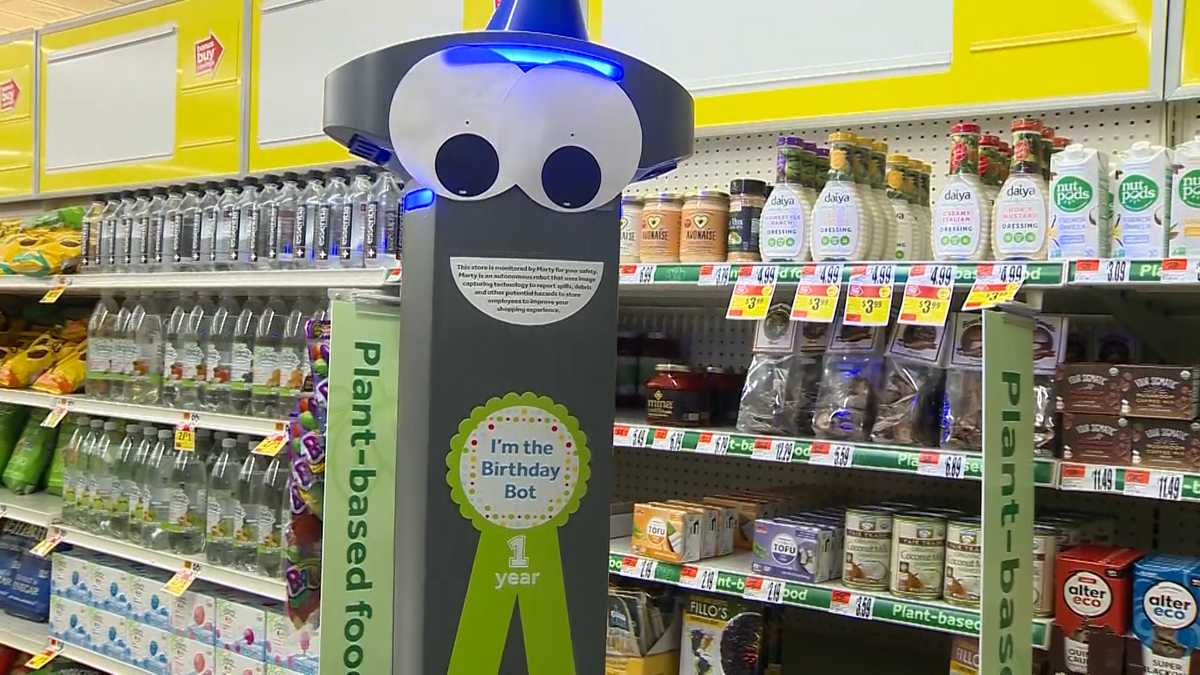
Reinforcement learning, a method of machine learning, makes use of the agent's interactions and its environment over an infinite number of time steps. A reinforcement-learning agent enters a situation st S, chooses an action at A(st) and receives a reward rt + 1 5R. The agent is placed in a new situation at the end of the time step.
Machine learning
There are a number of challenges associated with applying machine learning to reinforcement learning. The task the agent has to perform in reinforcement learning training is very dependent on what environment they are placed in. A simple game of chess, for example, can be trained in a simplified setting, while an autonomous car needs a more realistic environment. In this article we will examine some of these key issues when implementing machine learning for reinforcement in real-world applications.
Dopaminergic neurons
Dopaminergic neurons play a central role in the process of reinforcement learning. For researchers to understand how these neurons function, they must be able to comprehend both the neurophysiological wiring and the algorithms. Pavlov's famous experiment that showed dogs increased salivation after hearing a whistle is a great example of this process. This experiment is a classic example conditioned response, one the most fundamental empirical regularities of learning.
Architectures with actor-critic components
Actor-Critic architectures used in reinforcement learning are based on the assumption of greater success if a state is present. This assumption is not always fulfilled and can result in high variance in training. To prevent this, it is essential to have a baseline. Then, the critic (V) is trained to be as close to G as possible. The probability of an action increasing if the critic is absent will be due to the expected return, which can be non-linear.
Q-value
The Q-value of reinforcement learning is a function which represents the value a particular state, or action. The Q-value for picking up a package will be greater than the value for going north. Its value to go south is likely lower than its value to go north. This value is known as the "value function", and it represents the goodness or state of an action. Depending on context, multiple Q-values can be assigned to a single state.
Algorithms that are value-based
Recent research shows that value-based algorithms are more effective than traditional methods for reinforcement learning. These methods solve the cart-pole environment with fewer samples and are considered more reliable. However, the value of value-based algorithms is still not fully understood. Here are some examples of how they are used. They are more effective and produce better results. However, the results can be misleading. There are two important issues to be aware of.
Algorithms based on policy
Reinforcement-learning algorithms use a reward mechanism to assign values for different states of their environment. These reward systems are state-based and can be given to agents depending on their actions. The policy of a system determines which states, actions, and countries should be rewarded. The policy can either be immediate or delayed. It describes the behaviour of the agents and what actions should earn them the most rewards. This model can be used to solve problems such as reinforcement learning.
FAQ
Why is AI so important?
It is expected that there will be billions of connected devices within the next 30 years. These devices will include everything from fridges and cars. Internet of Things, or IoT, is the amalgamation of billions of devices together with the internet. IoT devices are expected to communicate with each others and share data. They will also be able to make decisions on their own. A fridge might decide whether to order additional milk based on past patterns.
It is estimated that 50 billion IoT devices will exist by 2025. This represents a huge opportunity for businesses. However, it also raises many concerns about security and privacy.
Are there potential dangers associated with AI technology?
Yes. There will always exist. Some experts believe that AI poses significant threats to society as a whole. Others argue that AI can be beneficial, but it is also necessary to improve quality of life.
AI's potential misuse is the biggest concern. If AI becomes too powerful, it could lead to dangerous outcomes. This includes autonomous weapons and robot rulers.
AI could also take over jobs. Many people worry that robots may replace workers. Others believe that artificial intelligence may allow workers to concentrate on other aspects of the job.
Some economists believe that automation will increase productivity and decrease unemployment.
How does AI affect the workplace?
It will change how we work. We can automate repetitive tasks, which will free up employees to spend their time on more valuable activities.
It will help improve customer service as well as assist businesses in delivering better products.
This will enable us to predict future trends, and allow us to seize opportunities.
It will enable companies to gain a competitive disadvantage over their competitors.
Companies that fail AI adoption are likely to fall behind.
Statistics
- While all of it is still what seems like a far way off, the future of this technology presents a Catch-22, able to solve the world's problems and likely to power all the A.I. systems on earth, but also incredibly dangerous in the wrong hands. (forbes.com)
- In the first half of 2017, the company discovered and banned 300,000 terrorist-linked accounts, 95 percent of which were found by non-human, artificially intelligent machines. (builtin.com)
- More than 70 percent of users claim they book trips on their phones, review travel tips, and research local landmarks and restaurants. (builtin.com)
- Additionally, keeping in mind the current crisis, the AI is designed in a manner where it reduces the carbon footprint by 20-40%. (analyticsinsight.net)
- The company's AI team trained an image recognition model to 85 percent accuracy using billions of public Instagram photos tagged with hashtags. (builtin.com)
External Links
How To
How to set Alexa up to speak when charging
Alexa, Amazon's virtual assistant can answer questions and provide information. It can also play music, control smart home devices, and even control them. It can even speak to you at night without you ever needing to take out your phone.
Alexa is your answer to all of your questions. All you have to do is say "Alexa" followed closely by a question. Alexa will respond instantly with clear, understandable spoken answers. Alexa will improve and learn over time. You can ask Alexa questions and receive new answers everytime.
You can also control connected devices such as lights, thermostats locks, cameras and more.
Alexa can also be used to control the temperature, turn off lights, adjust the temperature and order pizza.
Alexa to Call While Charging
-
Open Alexa App. Tap Settings.
-
Tap Advanced settings.
-
Select Speech Recognition
-
Select Yes, always listen.
-
Select Yes, you will only hear the word "wake"
-
Select Yes, and use the microphone.
-
Select No, do not use a mic.
-
Step 2. Set Up Your Voice Profile.
-
Select a name and describe what you want to say about your voice.
-
Step 3. Test Your Setup.
Say "Alexa" followed by a command.
Ex: Alexa, good morning!
If Alexa understands your request, she will reply. For example: "Good morning, John Smith."
Alexa won't respond if she doesn't understand what you're asking.
Make these changes and restart your device if necessary.
Notice: If the speech recognition language is changed, the device may need to be restarted again.